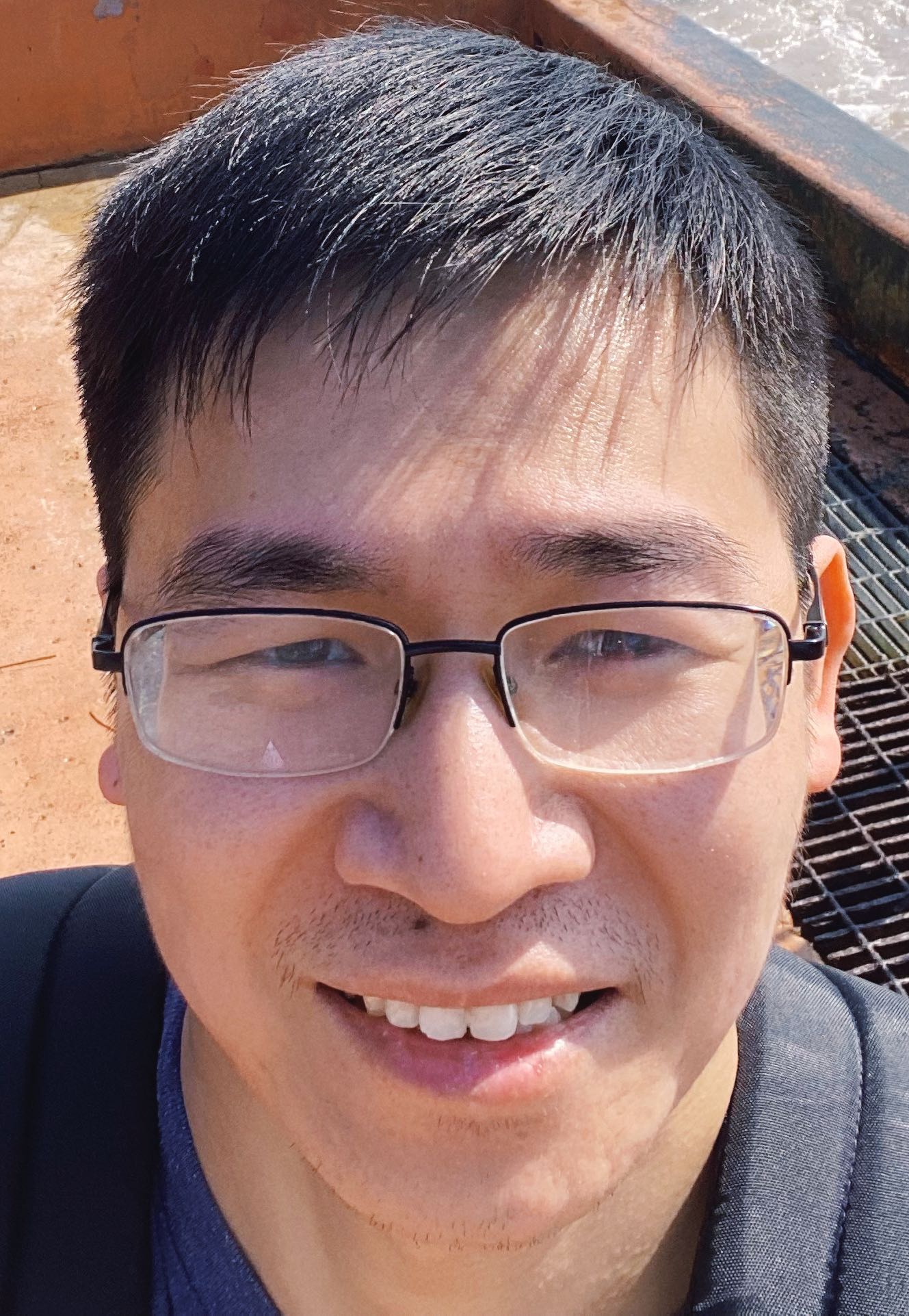
Chuxu Zhang
Assistant Professor
Email: chuxuzhang[at]brandeis[dot]edu
About Me
I am an Assistant Professor of Computer Science at Brandeis University. My general research interests center around artificial intelligence, machine learning, and data science. Recently, I have focused on developing effective, efficient, safe, and generative machine learning models and algorithms on graph data and multi-modality data (e.g., text and image). Besides, I apply machine learning to solve societal challenges in healthcare, social media, science, and others. Before joining Brandeis, I did my PhD study at University of Notre Dame (2017-2020), advised by Nitesh Chawla.
News
- Looking for multiple PhD students and interns (Spring 2025, Fall 2025).
- The workshop on Resource-efficient Learning at KDD'24: [Call For Paper], [Paper Submission Site].
- AC for NeurIPS'24, KDD'24
- Talk: Graph Machine Learning: Effectiveness, Efficiency, and Safety [slide]
- 03/2024 - Received Frontiers of Science Award from ICBS 2024.
- 02/2024 - Received NSF CAREER Award on graph machine learning! Thanks NSF, my excellent mentors and students:)
- Talk: Taming Networks in the Wild: A Holistic Learning Framework [slide]
- AC/SPC for AAAI'24, IJCAI'24, WSDM'24, CIKM'24, SDM'24, Track Chair for COLING'24
- 08/2023 - The workshop on Resource-efficient Learning at KDD'23: [Call For Paper], [Paper Submission Site].
- 07/2023 - Received an NSF grant on dietary recommendations.
- Talk: Towards Societal Impact of AI [slide]
- Selected as AI 2000 Most Influential Scholar Award Honorable Mention by AMiner.
- SPC for AAAI'23, WSDM'23, SDM'23, KDD'23, IJCAI'23, CIKM'23
- Selected as New Faculty Highlight at AAAI'23.
- Talk: Resource-efficient Graph Representation Learning [slide]
- 08/2022 - Received an NSF grant on drug trafficking network detection and intervention.
- 05/2022 - KDD'22 Tutorial: Towards Graph Minimally-supervised Learning
- Talk: Few-shot Learning on Graphs [slide] [survey]
Publications
Here are some recent publications. Please see my Google Scholar page for a complete list.
- ICML'24: From Coarse to Fine: Enable Comprehensive Graph Self-supervised Learning with Multi-granular Semantic Ensemble (Oral, Top 1.5% Paper)
- ICLR'24: Mitigating Severe Robustness Degradation on Graphs
- KDD'24: Graph Cross Supervised Learning via Generalized Knowledge
- KDD'24: Diet-ODIN: A Novel Framework for Opioid Misuse Detection with LLM-based Interpretable Dietary Patterns
- ICML'23: When Sparsity Meets Contrastive Models: Less Graph Data Can Bring Better Class-Balanced Representations
- ICLR'23: Chasing All-Round Graph Representation Robustness: Model, Training, and Optimization
- ICLR'23: Multi-task Self-supervised Graph Neural Networks Enable Stronger Task Generalization
- ICLR'23: Learning MLPs on Graphs: A Unified View of Effectiveness, Robustness, and Efficiency
- NeurIPS'22: Label-invariant Augmentation for Semi-Supervised Graph Classification
- NeurIPS'22: Co-Modality Imbalanced Graph Contrastive Learning
- KDD'22: Task-Adaptive Few-shot Node Classification
- KDD'22: Disentangled Dynamic Heterogeneous Graph Learning for Opioid Overdose Prediction
Students and Mentees
Current
Former
- Xiangchi Yuan (Brandeis MS, Next: PhD student at Goergia Tech)
- Zheyuan Liu (Brandeis BS, Next: PhD student at Notre Dame)
- Chunhui Zhang (Brandeis MS, Next: PhD student at Dartmouth)
- Erchi Zhang (Brandeis BS, Next: MS student at NYU)
- Jiele Wu (BIT BE, Next: PhD student at NUS)
- Qiannan Zhang (KAUST PhD, Next: PostDoc at Cornell)
- Qiang Yang (KAUST PhD, Next: PostDoc at UF)
- Yiyue Qian (Notre Dame PhD, Next: Research Scientis at Amazon)
- Qianlong Wen (Notre Dame, Next: PhD student at Notre Dame)
- Yijun Tian (Notre Dame PhD, Next: Research Scientis at Amazon)
- Zhichun Guo (Notre Dame PhD, Next: Assistant Professor at Emory)
- Mingxuan Ju (Notre Dame PhD, Next: Research Scientis at Snap)
- Zhongyu Ouyang (Notre Dame, Next: PhD student at Notre Dame)
- Jianan Zhao (BUPT BE, Next: PhD student at Mila)
Teaching
- Artificial Intelligence
- Deep Learning
- Graph Machine Learning
Last update in 06/2024
|